Steps towards being more FAIR
Who wouldn't want to be FAIR? Many data archives are now striving to become more FAIR, but the task can be challenging. And researchers may have to consider for themselves whether the data management and curation services available to them have been implemented with consideration for the FAIR principles. This spring, the Finnish Social Science Data Archive has made a number of changes to its metadata that should make our services more FAIR.
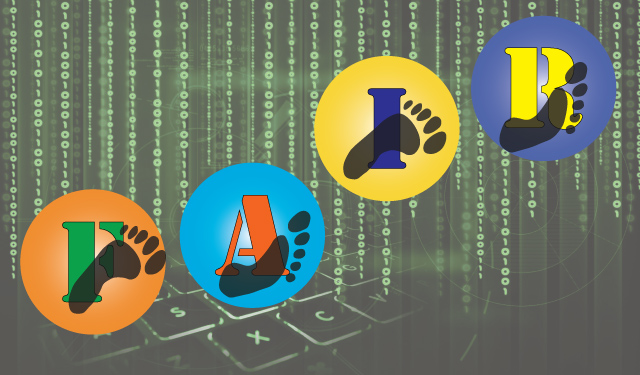
The European Open Science Cloud (EOSC) is an infrastructure that promotes open science. EOSC-Nordic is a project that aims to promote EOSC-relevant goals in the Nordic and Baltic region. One of these goals is to make recommendations on implementing the FAIR principles.
FSD participates in drawing up these recommendations. To this end, the project has carried out an extensive landscaping task. The aim is to get a bigger picture of the current state of data repositories when viewed in terms of FAIR maturity criteria.
FAIR is certainly an acronym already familiar to anyone working in the field of managing or opening research data. Perhaps so familiar that it is referred to almost automatically, denoting that the goal is to be either FAIR or even more FAIR. The general understanding is that FAIR is a desirable state of affairs, one that helps to promote transparency and re-use of research data. The sixteen FAIR Guiding Principles, which address the findability, accessibility, interoperability and reusability of research data, are not as well-known as the general objective.
Although the principles themselves seem quite understandable at first sight, there is a rather technical framework behind them. Implementing the principles as a concrete part of repository's services goes partly beyond normal data management tasks. The necessary information content gets created at the repository but publishing it in a FAIR way is a separate challenge. The FAIR principles aim at enhancing the ability of machines to automatically find and use research data. In simpler terms, this means that the machine can find data and understands how to access, process and reuse them. But the way that machines and humans interpret information are rarely uniform.
A common mistake is to assume that FAIR data and open data are synonymous. These certainly are related, but access to data (or the method or code developed to produce it) may be very restricted, but still completely FAIR. What is essential is that the machine and data user know about the existence of data, the conditions under which the data can be accessed and what using the data requires. How these are defined may vary by discipline or research community. They need not be generally binding.
How to measure FAIR maturity?
At FSD, we have curated and described research data for two decades. We have been actively involved in the development of metadata models and vocabularies. We have promoted the openness and discoverability of data. The metadata descriptions which we produce are rich and openly available under the CC BY 4.0 license. We know that these descriptions are harvested into several common catalogues. These are all areas that FAIR thinking seeks to promote.
When an organisation sets a goal to become more FAIR, the next logical question should be "How can this be measured?" Evaluating FAIRness is not straightforward because the principles are indicative in nature, do not require a narrowly defined set of standards to be used, and as said, practices and definitions for FAIR may vary between scientific communities. FAIR is also not "either or" but rather "to what extent".
The Maturity Indicator Authoring Group has developed 22 maturity indicators to help evaluate FAIRness. These tests can be applied to any digital object on the web and the evaluator performs the tests fully automatically, resulting in information on how many tests were passed successfully and why. The findings are machine-interpreted, and it is not even the point to provide human interpretation.
Definition of maturity in the EOSC-Nordic project
In the EOSC-Nordic project, the level of FAIR maturity in is defined based on these evaluations. In the landscaping stage, a large number of Nordic and Baltic data repositories were selected for maturity review. Definitions for a data repository were very broad; any organisation providing access to some kind of research data was qualified. The next step was to identify and evaluate at least ten digital objects from each repository. This was an arduous step. Locating individual datasets was not at all as unambiguous a task as one might think. Many were dropped off the sample at this point, for example because there were no open metadata available.
Based on the test results, average "FAIR Scores" were calculated. Far-reaching conclusions cannot be drawn in the light of these figures, but they do provide a big picture on how well the repository is able to present its holdings in a machine-readable form. That is, how well a machine can "understand" what type of an actor it is interacting with, and what data are available to it.
Contradictory feelings about the first results
In the evaluator, the FAIR principles have been broken down into component tests for findability, accessibility, interoperability and reusability. They emulate how a computer approaches an object. The tests look for machine-readable (meta)data and characteristics that support the qualities mentioned in the principles. For example, do the (meta)data have a unique and permanent identifier, can they be accessed using well-known retrieval protocols, are open and machine-readable vocabularies used to describe (meta)data, and are licenses and terms of use explicitly defined.
We know that FSD has a lot of high-quality metadata and they richly describe our diverse collection of datasets. We know that we are at least fairly FAIR.
Therefore, undeniably, it was a disappointment that the first maturity level tests gave results that only barely exceeded the minimum level. We got 4/22 points. No reason to raise a toast.
Obviously we immediately noticed that several tests failed because the evaluator did not recognise the way that we had chosen to express what was sought in the test. These were, for example, the license information provided for the metadata and data descriptions in machine-readable XML format. Neither of these were identified by the evaluator. For a long time, we have provided clear terms of use for both data and metadata - and licensing of metadata is still relatively rare across repositories. And this practice should be well in line with the FAIR principles1.
A closer look gives hope for the better
When we looked more closely, we noticed that in each record the metadata license was described in human language, in standard HTML markup. The evaluator completely ignored it and stormed ahead totally unaware that we had this information available. When we expressed the same information in a standardised way using Creative Commons Rights Expression Language, which has been developed to allow machines read licensing information, the test passed successfully.
The same was true for the XML file - it was not understood by the machine because the test had not been developed to accommodate this type of expression. However, this does not mean that anything was necessarily wrong. If our way had matched to some community-specific definition of how to describe FAIR metadata in a machine-readable way, the test could have been adapted to it.
We looked at each individual test result similarly. We found that the machine had difficulty interpreting our high-quality data descriptions. Based on this discovery, the solution was obvious - we enriched our metadata by embedding linked data that described the dataset. We used JSON-LD format and schema.org datatypes. The change is not visible in any way to a researcher who visits Aila to browse or search the holdings. This information is there only for the machine.
This resulted in a considerable improvement in the maturity evaluation. In the latest evaluation, we scored 17/22. (Popping the bubbly awaits a time when it is safe for the entire staff to gather in the office.)
In the EOSC-Nordic project, we have already suggested that some aspects of maturity evaluation could be measured differently. On the other hand, generic tests can only be flexible up to a certain limit. Some results should be interpreted in the spirit of FAIR, and not as rigidly as in the test. Thus, for example, we interpret that the URN which we assign to the datasets is a persistent identifier, even though it was not recognised by the tests measuring identifier persistency.
Lessons learnt
There are many reputable data archives around the world. Many of them have been at the forefront of reuse and machine readability of research data for decades. Therefore, weak performance in an evaluation may, as a first reaction, lead to curling up in defence like a hedgehog. It is clear that competence cannot be condensed to a simple maturity level test. The evaluation has its known limitations regarding content and technology.2
However, it is still beneficial to openly consider how the job well done is visible to a machine, not only the customers. It is safe to say that the requirements for machine readability will increase. Many new services are built on this assumption.
A majority of the necessary changes are essentially quite simple. They can be carried out by repurposing the metadata which has already been produced and by using some of the many existing metadata schemas. The difficulty factor increases if these operations are not supported by the software platform used to run the data catalogue. The road to a more FAIR tomorrow runs via workspaces of both a content specialist and a technical specialist.
The goal should not be set at a minimum level. Promoting openness of data also contributes to success in some tests. Many tests can be passed by providing very little, in some cases even completely irrelevant metadata. But the emperor who chooses this path has no clothes.
At FSD, we knew that we can be FAIR and we wanted it to show up in the evaluation as well. We also wanted the changes we made to genuinely enhance the (re)usability of our holdings. Therefore we provide basic information about the dataset and authors, date of publication, terms of use, and the identifiers given to the material in machine readable linked data - everything that we think the machine needs to be able to tell the end user about what we have to offer.
1See principle R1.1. (Meta)data are released with a clear and accessible data usage license.
2The evaluator uses only the REST API - in practice this means that the digital objects must be testable over HTTP. The evaluator does not support protocols such as OAI-PMH or SOAP.
Text and illustration (CC BY 4.0): Tuomas J. Alaterä (OpenClipart-Vectors - Pixabay and Pxfuel)
About the author
Tuomas Alaterä works at FSD as an IT Services Specialist. Her areas of expertise include communication, open science, online services and digital long-term preservation. In the EOSC-Nordic project, he is involved in the tasks that aim to enable FAIR data practises and repository certification.