Anonymisation and Personal Data
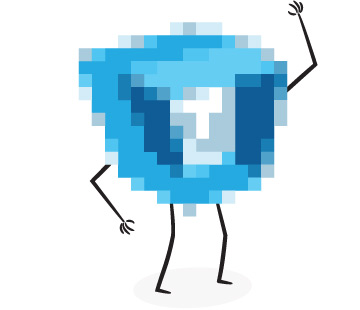
What is personal data?
According to the definition given in the General Data Protection Regulation (GDPR), 'personal data' means any information relating to an identified or identifiable natural person. A natural person is considered identifiable if they can be identified, directly or indirectly, in particular by reference to an identifier such as a name, an identification number, location data, an online identifier or to one or more factors specific to the physical, physiological, genetic, mental, economic, cultural or social identity of that natural person. ( EU General Data Protection Regulation Article 4 (Opens in a new tab) , Paragraph 1). By this definition, when it comes to research data, personal data are not limited to information relating to research participants. Research data may also contain identifiers relating to research subjects' family and friends or other third parties. Identifying information relating to these persons also constitutes personal data.
There are no limitations regarding the nature and character of personal data. Any information related to a natural person may be personal data. This includes statements, opinions, attitudes and value judgments. Personal data may be objective or subjective. Whether the information is true or verifiable or not is of no consequence here. The information may refer to an individual's private or family life, health, physical characteristics, professional activities, and economic or social behaviour.
What kind of information constitutes identifiable data?
Personal data are any kind of data that may be used to identify a natural person or a cluster of persons, such as individuals in the same household. Identification can occur on the basis of one or more factors specific to the physical, psychological, mental, economic, cultural or social identity of an individual or individuals. Data that are not directly about people can also be personal if they contain identifiers. An example of secondary personal data could be fire department information on the occurrences of fires, which may include addresses. (Elliot et al. 2016.)
Information that is sufficient on its own to identify an individual includes a person's full name, social security number, email address containing the personal name, and biometric identifiers (fingerprints, facial image, voice patterns, iris scan, hand geometry or manual signature). These type of data are called direct identifiers .
Other information that may be used to identify an individual fairly easily include a postal address, phone number, vehicle registration number, bibliographic citation of a publication by the individual, email address not in the form of the personal name, web address to a web page containing personal data, unusual job title, very rare disease, or position held by only one person at a time (e.g. chairperson in an organisation). A rare event can also reveal the identity of an individual. The Finnish Social Science Data Archive (FSD) calls these types of information strong indirect identifiers .
At FSD, strong indirect identifiers also include the types of codes that can be used to unequivocally identify an individual from among a group of individuals. These include, for instance, a student ID number, insurance or bank account number, IP address of a computer etc.
Indirect identifiers (or quasi-identifiers) are the kind of information that on their own are not enough to identify someone but, when linked with other available information, could be used to deduce the identity of a person. Background variables and indirect identifiers include, for instance, age, gender, education, status in employment, economic activity and occupational status, socio-economic status, household composition, income, marital status, mother tongue, ethnic background, place of work or study and regional variables. Indirect identifiers relating to region of residence include, for example, postal code, neighbourhood, municipality, and major region.
Date can also be an indirect identifier. Date of birth is the most common example, but dates of death and dates of newsworthy events may also be indirect identifiers in research data when combined with other information. In health and medical research, treatment and sampling dates may also occasionally be indirect identifiers when linked with other information.
Pseudonymous data are also taken to be personal data. These include data from longitudinal studies where participants have a case ID instead of a name or social security number, but the research team has a key that can be used to connect the data to research participants.
Processing research data containing identifiers
Identifiable data may be used for scientific research when the use is appropriate, planned and justified, and when there is a legal basis for processing the data (e.g. consent of participants or research carried out in the public interest).
From the point of view of research participants, processing personal data constitutes the risk of confidential information relating to them being revealed to outsiders (for instance, people close to them, employers or authorities). Therefore, personal data processing must be planned thoroughly and executed carefully. Data protection must not be jeopardised, for example, by careless preservation or insecure digital transfers. You can adapt the various guarantees presented in these Data Management Guidelines, including data minimisation, pseudonymisation and anonymisation, for your purposes when processing personal data. Anonymisation is one way of making the data available for sharing and reuse. If necessary, the data can be further protected by administrative and technical data security solutions.
More information on data security
Terms to understand
Anonymous data: An individual data unit (person) cannot be re-identified with reasonable effort based on the data provided or by combining the data with additional data points. Completely anonymous data do not exist, but with well-executed procedures one can achieve a result where individual persons cannot be identified with reasonable effort. Anonymisation refers to the various techniques and tools used to achieve anonymity.
Pseudonymous data: An individual data unit cannot be re-identified based on the pseudonymised data without additional, separate information. Pseudonymisation refers to the removal or replacement of identifiers with pseudonyms or codes, which are kept separately and protected by technical and organisational measures. The data remain pseudonymous as long as the additional identifying information exists.
De-identification: Removal or editing of identifying information in a dataset to prevent identification of specific cases. De-identification often refers to the process of removing or obscuring direct identifiers (Elliot et al. 2016).
De-anonymisation: Re-identification of data that are classified as anonymous by combining the data with information from other sources. If anonymous data are de-anonymised, the data were not truly anonymous to begin with, technology has advanced, or more information on the individuals has become available elsewhere. This is why it is good practice to re-assess the robustness of the anonymisation periodically (the so-called residual risk assessment ).
Special categories of personal data: Personal data specified in data protection legislation that reveal racial or ethnic origin, political opinions, religion or philosophical beliefs, trade union membership, data concerning health, or sexual life or orientation. These special categories also include genetic and biometric data for identifying a natural person.
Minimisation: Only the minimum amount of personal data necessary to accomplish a task (e.g. research) should be collected. Personal data must not be collected just in case they might be useful in the future. There has to be a clear, specified need for collecting the personal data.
Storage limitation: Personal data that are no longer needed to conduct the research should be erased as soon as possible. For example, names, addresses and other similar identifiers should be removed immediately after they are no longer necessary to carry out the research. If social security numbers were used to link data, they should also be deleted when they are no longer needed. Storage limitation reduces risks related to personal data processing.
When are data anonymous and when pseudonymous?
Data are anonymous if characteristic attributes (e.g. combinations of certain indirect identifiers) pertain to more than one person and a data subject cannot be identified with reasonable effort.
The EU data protection regulation (GDPR) defines anonymous data in a functional manner, as part of an activity:
To determine whether a natural person is identifiable, account should be taken of all the means reasonably likely to be used, such as singling out, either by the controller or by another person to identify the natural person directly or indirectly. To ascertain whether means are reasonably likely to be used to identify the natural person, account should be taken of all objective factors, such as the costs of and the amount of time required for identification, taking into consideration the available technology at the time of the processing and technological developments.
Source: EU GDPR, Recital 26 (Opens in a new tab)
When data are anonymous, individual research participants or third persons cannot be identified based on indirect identifiers or by combining the data with information available elsewhere. New data on the same research subjects cannot be added to an anonymous dataset. For the data to count as anonymous, anonymisation must be irreversible.
Pseudonymous data do not allow the identification of a data subject without the use of separately stored additional information. When data are pseudonymised, unique records are replaced by consistent values either derived from the original values or independent of them so that specific data subjects are no longer identifiable. In addition, information on the original values and techniques used to create the pseudonyms should be kept organisationally and technically separate from the pseudonymised data. Organisational measures refer to the protection of physical environment and documented access control. Technical measures include, for example, secure data storage and encryption. (Tarhonen 2016.)
Data are not pseudonymous if a specific data subject is identifiable from the data solely without additional information (ibid.). This could happen when indirect identifiers and exceptional records enable identification, even if social security numbers and other direct identifiers are stored separately and securely. Pseudonymisation has also been unsuccessful if an outside person is able to determine the original values based on the pseudonyms. This may happen if the original identifiers are only redacted partially, for instance, "Arja Kuula-Luumi" is changed to "Arxx Kuxx-Luxx" or the social security number 123456-789E is changed to 123456-XXXX. The first part of a Finnish social security number denotes an exact date and year of birth and is thus in itself a fairly strong indirect identifier. Such measures should be taken in pseudonymisation that outside persons have no possibility of determining the pseudonymised personal data.
Pseudonymous data become anonymous when separately kept identifying information (decryption key, personal data and information on the techniques used to pseudonymise the data) is destroyed. If you cannot dispose of the separately kept personal data, you can make pseudonymous data anonymous by destroying the decryption key and information on the pseudonymisation processes, and by re-arranging the data, for example, according to new, randomised case IDs. The data are anonymous if they cannot be linked to the original personal data with reasonable effort.
For instance, research data of a longitudinal study remain identifiable for as long as the research group has the decryption key to the personal data of the research subjects. The data will not become anonymous even if the decryption key is coded twice ('double coding'). However, coding and double coding as well as pseudonymisation in general are useful guarantees to prevent the use of identifiers in analyses. Coding and double coding are often used in medical sciences.
Further information concerning pseudonymisation and techniques for its implementation can be found, for instance, on the report of the European Network and Information Security Agency (ENISA): Recommendations on shaping technology according to GDPR provisions. An overview on data pseudonymisation (Opens in a new tab) (November 2018).
Minimisation, or how to collect only the minimum amount of personal data necessary
In principle, to reduce later anonymisation needs, one should avoid collecting data that are unnecessary, unnecessarily detailed, or insignificant for the research. Which background information is needed about research subjects and how detailed this information has to be should be considered already at the planning stage of data collection. The formulation of questions can affect the level of detail in the responses. Controlling the data collected is easier in quantitative research compared to qualitative research due to the ability to use categorised response alternatives.
One should bear in mind that the GDPR also prohibits collecting unnecessary personal data. If you are to collect data belonging to the special categories of personal data, special consideration in data collection planning is required. The special categories of personal data refer to personal data that reveal racial or ethnic origin, political opinions, religion or philosophical beliefs, trade union membership, data concerning health, or sexual life or orientation. These special categories also include genetic and biometric data for identifying a natural person.
Below are some tips for quantitative and qualitative datasets from the perspective of data minimisation.
Quantitative datasets
- Do not collect data about something that is exceptional in the population. If you plan to obtain information regarding a rare subject, this information should be collected in a categorised or coarsened form to avoid unique observations. In categorising variables, utilise existing social classifications. Examples are available e.g. on the website of Statistics Finland (Opens in a new tab) .
- If you wish to minimise personal data, do not utilise open-ended questions, as you will not be able to control the content of the responses. If you still want to use open-ended questions, consider how to formulate the questions so that you will be better able to control the obtained data. Do not use open-ended questions to collect background information, such as education or occupation – use categorised response alternatives instead. Consider the information obtained with an open-ended question in light of its usefulness and usability in future research. For instance, open-ended responses of the type "other, please specify" often produce unique or rare information that can be used to identify respondents.
- The following information, among others, should be collected using categorised alternatives: occupation, income, employment status, education level, nationality, and number of children. All values do not always have to have categories; it is possible to categorise only the extreme values (e.g. number of children: 0; 1; 2; 3; 4 and over).
Qualitative datasets
- If it is possible given the nature of the data collection situation, you can remind research participants before data collection (e.g. before an interview) that they should avoid mentioning personal names, exact dates, workplace names or detailed information concerning third persons. People are surprisingly good at anonymising events and their experiences.
- Think carefully which background information you wish to collect from research subjects and how to collect that information. Background information can be collected using a structured form, which is a good way to avoid free-form introductions by interviewees that often contain identifiers. In categorising background information, utilise existing social classifications. Examples are available e.g. on the website of Statistics Finland (Opens in a new tab) .
- During data collection (e.g. in an interview), do not ask specifying questions that will very likely produce responses requiring heavy anonymisation measures (No: "Could you tell us which workplaces your father and mother have worked in during their lives...").
Anonymisation principles
In the international literature of the field, anonymisation is defined as a broad umbrella concept containing different approaches such as access control and statistical anonymisation (Elliot et al. 2016). According to the GDPR's definition, access control is considered a safeguard but not anonymisation as such. Here we focus on data-oriented anonymisation measures that aim to remove all information from a dataset that could render it possible to identify individuals.
There is no single anonymisation technique suitable for all types of data. Anonymisation should always be planned case by case, taking into consideration the data features, environment and utility.
Data features refer to the age and sensitivity of the data, number of data subjects as well as how specific the data contents are (Elliot et al. 2016). Data environment refers to the context in which the data are used: who uses the data, when and where? What external data sources are available? Data environment also includes the physical storage of data . In assessing data utility, one must consider how to balance data utility and anonymisation so that the data remain as usable as possible in statistical or qualitative research after anonymisation.
You should plan anonymisation carefully and document all relevant anonymisation techniques and processes along with the rationale for them. You should reserve time for anonymisation, and anonymisation should be considered already during data collection, as careful planning will significantly reduce the resources needed for anonymisation. Researchers should consider the following factors concerning anonymisation beforehand:
- Ensure that data are collected according to the principle of data minimisation.
- Decide who plans and carries out the anonymisation and at which point.
Anonymisation planning
An anonymisation plan should include a description of the anonymisation measures and an evaluation of the disclosure risk of data subjects' personal data. The anonymisation plan also works as documentation on how the data have been processed. This information is important with regard to, for instance, colleagues in a collaborative research project or archiving the dataset for reuse. The GDPR also requires documentation on decisions related to personal data processing. Drafting an anonymisation plan can be started already during data collection.
The anonymisation plan should include the following information: creator(s) of the plan, person(s) carrying out the anonymisation, features in the data that have an impact on anonymisation, assessment of the disclosure risk of respondents' personal data, and anonymisation techniques used along with the rationale for using them. Finally, you can also assess the possibility of identifying persons in the data after anonymisation and the need for residual risk assessment in the future.
Below are FSD's examples for anonymisation plans for quantitative and qualitative datasets (in Finnish). A template to facilitate writing an anonymisation plan is available in English.
- FSD's Anonymisation plan template PDF
- Example of an anonymisation plan for a quantitative dataset (in Finnish) PDF
- Example of an anonymisation plan for a qualitative dataset (in Finnish) PDF
The first step in anonymisation, discussed in the following section, is charting the features of the data. The most important factors to take into consideration in qualitative and quantitative research data are presented in the figure below.
1. Population and sampling
Who were the target population of the study and how was sampling conducted? How many people belonging to the population were included in the sample? What is known about the population beforehand (e.g. distribution of gender and age)? Does the target population share a rare feature or phenomenon?
Population is the target of data collection, and the sampling method describes how units of observation are included in the data. When planning anonymisation, you should first consider whether the population and the sampling method might reveal exceptional or unique information on the research subjects. The population may be clearly defined and identifiable from the outside, such as city councillors in Tampere in 2009, or a random group not determinable from the outside, such as Finnish people who have experienced sexual harassment. The size of the population is important, because the smaller the population or the phenomenon under observation, the greater the possibility of being able to identify an individual person.
In connection with the population and sampling, one should consider how random belonging to the population and being selected for the sample are in relation to a larger scale, for instance, the population of the area. Examples follow.
Total population sample: An invitation to participate in the study is sent to all persons belonging to the target population, for example, all parents of prematurely born children under the age of one in Finland, or all residents of a specific municipality who are at least 18 years old. Because all persons belonging to the target population are included, it is possible to determine beforehand that a person may be included in the data.
Random sample: An individual belonging to the target population has a smaller probability of being included in the data than in a total population sample because only some of the individuals belonging to the population, e.g. every 50th individual, are selected in the sample.
Self-selected sample: It cannot be determined beforehand who will participate in the study, for example, via a link on the Internet. However, studies with a self-selected sample usually target persons who have experience about the phenomenon under observation. Hence, the nature of the observed phenomenon affects how likely it is that someone can be determined to be included in the data. Consider, for example, experiences of managing an organisation vs. experiences of health care services.
Irrespective of the population or the sampling technique, it is always important to examine what kinds of direct or indirect identifiers the data contain and to see if there are any exceptional or unique observations.
You should also pay attention to the response rate because it indicates the probability of an individual to be included in the data. This is particularly important in assessing the anonymity of total population data. The higher the response rate, the higher the likelihood of an individual being included in the data.
Information on how the data were collected, i.e. sampling and selection criteria, should not reveal the identity of research subjects. Disclosure risk is particularly notable if a researcher selects the study participants from his or her own social circle using snowball sampling or from an area with a small number of inhabitants.
2. Content of the data
In relation to the anonymisation of data content, you can ask:
Chart the information that could be used to identify a person in the data. Identification may be possible based on a direct identifier or by combining indirect identifiers with other indirect identifiers or with information available, for instance, on the Internet. More information in the section "What kind of information constitutes identifiable data?" . You can even try to identify data subjects yourself and see if it is possible by combining different kinds of information. Note that identifiers may also appear elsewhere than in individual variables of quantitative data or background information shared in a qualitative interview. In a quantitative dataset, identifiers may be found in all open-ended responses, and a qualitative dataset may contain identifiers just about anywhere.
The first anonymisation measure is usually to remove direct and strong indirect identifiers from the data (see the Identifier type table ). However, removing direct and strong indirect identifiers is rarely sufficient to make the data anonymous. The number of indirect identifiers and their level of detail affect the anonymisation choices. The variables that contain information about people should always be examined in relation to one another. Combining only a few background variables may render it possible to identify an individual. For instance, combining gender, age, municipality of residence and income may reveal identities of high-income persons residing in a small municipality.
Information appearing in a dataset regarding third parties also has to be taken into consideration during anonymisation. In some cases, the respondent's identity can be determined based on information about a third person, and in other cases a third person's identity can be determined based on the respondent's information. Public figures who are referred to on a general level do not have to be anonymised. If you are unsure whether you should redact the name of a public figure from the data, you can assess whether the information in the data is public knowledge and of such social interest that it should be left in the data.
We were never really very religious even though my aunt was one of the first women to be ordained as priest in Finland.
Information on the respondent's aunt being one of the first female pastors may increase the risk of disclosure of the respondent's identity, since information is publicly available on the first female pastors. On the other hand, the first ordination included 94 female pastors, which is a relatively high number. Whether the information should be redacted or not depends on the other background information regarding the research participant.
Walking around town I often used to bump into Member of Parliament Satu Hassi and sometimes even exchanged a few words with her.
The name of a Member of Parliament can be left in the data if information that would be private in nature is not disclosed and if the possible disclosure of the municipality does not jeopardise the respondent's anonymity. Based on publicly accessible information, the municipality in question could be determined to be either Tampere, which is Hassi's city of residence, or Helsinki, where she works.
The exceptionality of an observation is based on either an individual piece of information or cumulated information about a respondent. An observation is exceptional if its prevalence in the population is small. Exceptional information has to be anonymised, especially if the respondent can be identified using information from other sources.
The following figure illustrates the assessment of the information between a respondent and the population. Many types of differing information is obtained about research participants, such as having a dog named Peppi or a stepfather, suffering from depression, or being a blogger or a competing athlete. Pertaining to the information obtained from research participants, one should assess whether the information is common or exceptional in the population.
If a 2,000-respondent sample is drawn of the Finnish population, observations regarding stepfathers or depression are not exceptional in the target population, i.e. the whole Finnish population. More exceptional is owning a dog named Peppi, competing as an athlete, and blogging. Depending on the other information available on the research subjects, the aforementioned observations may have to be anonymised. If the population is a clearly defined group of people, such as pupils in a small elementary school, observations concerning stepfathers, not to mention blogging or owning a dog named Peppi, will very likely make it possible to identify a person.
Depression is an example of an observation that usually does not require anonymisation because the information is invisible by nature. In other words, it is not necessarily noticeable to outsiders and such information is often only shared to the people closest to the individual. An observation regarding depression may, however, make it possible to identify a person if it appears, for instance, in a workplace survey and the person has spent a long time on a sick leave due to depression.
Thus, exceptional information does not automatically constitute a disclosure risk because such information is not always publicly available.
A population-level dataset reveals that one respondent suffers from catoptrophobia, which is a persistent fear of mirrors.
The observation is exceptional but not necessarily identifiable; drawing a connection to a specific person is difficult because information on persons suffering from catoptrophobia is not publicly available.
Exceptional information in population-level studies may include, for instance, a rare occupation or position at an organisation or as a high-ranking politician. Exceptional information may also include high income or wealth, diseases, competing in a specific sport, hobbies, or participating in an event that has been on the media.
In population-level data, anonymity can effectively be enhanced by coarsening information regarding region.
Golf as a hobby is not an exceptional observation at the population level.
However, a person playing golf who resides in the Pirkanmaa region and works as school principal is very likely an exceptional observation. It is possible that only a couple of principals play golf in that region. A principal's golf hobby may also have been mentioned in an interview for a local paper.
In some cases, the phenomenon under observation is in itself exceptional, and one has to ensure the sufficient size of the target population from which the sample is selected. For example, when examining professional athletes competing in winter sports, the regional coverage of the study has significance for anonymity because the number of athletes differs between municipalities, regions and even countries. In terms of researching an exceptional phenomenon, the larger the geographical region of the study, the more anonymous the data.
In practice, the values each respondent provides in a quantitative survey as well as qualitative datasets may produce unique sets of information that do not exist anywhere else in the world. If the information was accessible from the outside, the persons could be identified. However, it is difficult to draw connections between persons and their opinions and attitudes in survey studies because people themselves also forget the responses they provide. In addition, when people describe a past event in an interview, they may recall and tell the story completely differently after, say, a year. The important thing is to assess whether it is possible to identify a person by connecting different types of information within the dataset or by connecting the data to information available in other sources.
Information in a dataset is sensitive if it contains categories of personal data specified in data protection legislation concerning racial or ethnic origin, political opinions, religion or philosophical beliefs, trade union membership, data concerning health, sexual life or orientation, or genetic or biometric data for identifying a natural person. Other information may also be sensitive by nature. Sensitivity can be assessed by, for instance, considering whether the phenomenon is taboo or how much the disclosure of the information could provide harm to a person, organisation or other unit of observation.
Examples of other types of sensitive information include: descriptions of criminal events (e.g. domestic violence), criticism against other persons, detailed descriptions of third persons' personal lives, or trade secrets.
3. Dataset age
The age of the data has an impact on the need for anonymisation. The older the data, the more difficult it is to identify individuals, because the information changes over time. Information that is over 100 years old or concerns deceased individuals does not have to be protected.
4. Information on respondents available in other sources
For successful anonymisation, information included in the data should be considered together with information available in other sources. Data must be processed in a way that no individual can be identified, even when using information obtained from other sources.
The information in a dataset should be considered in relation to four different types of information (Elliot et al. 2016):
- information and research data about the same target population available elsewhere
- publicly available information (e.g. public registers, social media)
- local knowledge (what residential locations look like and what goes on in the area)
- personal information about other people (what do I know e.g. about my neighbours)
The more likely it is that information is commonly known or available elsewhere, the more controlled the information in the data has to be. The following contain examples of different datasets and information sources that could be combined with them in order to identify respondents.
a. The data examine Finnish people's career paths. Outside information on Finnish careers is available on the Internet, e.g. on LinkedIn, social media services such as Facebook, and personnel information on websites of organisations.
b. The data examine Finnish people's meal patterns. Outside information is not easily available, although an examination of a person's meal pattern can produce fairly detailed information about his/her everyday life. The relevant thing to consider is how it would be possible to obtain information concerning other people's meal patterns (not very easily or the information would not differ very much from person to person).
c. The data examine neighbour relations among Finnish people and Tanzanian people. Here one should consider the cultural differences between Finland and Tanzania: to what extent do people acquaint with their neighbours? Finnish interaction with neighbours can be very minimal, whereas it can be more active in Tanzania. Thus, anonymisation of the Tanzanian data would probably require more effort than the Finnish data.
If reports or publications have already been published based on the data, consider how detailed the information about the data is that has been provided in the publications.
In connection with one quantitative dataset, the researchers decided to anonymise a variable denoting municipality of residence by removing the name of the municipality and leaving its numerical value in the data (1, 2, 3...) e.g. for multilevel analyses. Afterwards they noticed that a publication made previously based on the data included the number of respondents in different municipalities. The anonymisation had thus failed because the names of the municipalities could be de-anonymised based on the number of respondents for each of the values.
To summarise, information obtained from external data sources is of considerable significance in anonymisation. Latanya Sweeney (2000) found out in her study that 87% of Americans are likely to be uniquely identified based on their date of birth, gender and a 5-digit ZIP code, which appear in voter registration lists containing personal and regional information on people who have voted. Similarly, over half of the population in the United States (53%) are likely to be uniquely identified by gender, birth date and place, i.e. city, town or municipality of residence (ibid.).
5. Usability vs. anonymity
Anonymisation always reduces information in the data. The degree of anonymisation affects the usability of the dataset and the accuracy of the results. In ideal situations, only changes that are as small as possible are made to the data, avoiding any edits to variables that are the most significant for research. Most often it is easier said than done.
Successful anonymisation requires that the person processing the data recognises which information is of significance for current and future research and which information has less importance. Complete removal of values with identifiers should only be done with less significant information. Sometimes, for instance, removing open-ended variables from quantitative datasets drastically reduces the amount of exceptional or unique information in the data. Numerical or categorical variables are also usually easier to process and use in quantitative research than open-ended responses. That said, significant variables, such as age, often have to be edited as well to achieve anonymity.
If you want to leave information regarding municipality of residence in the data, other background information about the person has to be anonymised. This means that occupation, workplace, education, age, etc. may have to be coarsened to a level sufficient to prevent identification. If it is important in terms of the content of the data to leave in information regarding the research subject's occupation and age, information concerning region of residence should be coarsened (instead of municipality of residence, indicate major region and/or type of municipality), and the need to edit other background information should also be assessed.
Assessing the robustness of anonymisation
As Elliot et al. (2016) point out, "[a]nonymisation is not an exact science," so determining the sufficient level of anonymisation may sometimes prove problematic. However, you can use the following questions to assess your choice of anonymisation technique and the robustness of the outcome (adapted from EU's article 29 working group: Opinion 05/2014). If you answer the first two questions in the negative and there is a very small chance of inference, the anonymity of the data is in good order.
- Singling out an individual: Can you still single out any individual in the data after anonymisation?
- Linkability: Can you link records relating to an individual to another dataset or information from external sources and thus identify the individual?
- Inference: Can you infer that certain information concerns a specific individual? Can you infer the original values of altered or removed values?
NB: Due to the constantly increasing extent of publicly available information, it is important to regularly assess whether a once anonymised dataset continues to be anonymous ('residual risk assessment').
Anonymisation of quantitative data
Practical tips for anonymising quantitative data:
- Utilise the syntax in your statistical software in anonymising your data.
- Anonymise numerical and categorical variables first and only then open-ended variables, because the strategy adopted for the anonymisation of numerical variables will often outline the strategy for the anonymisation of open-ended variables as well.
- Mark the anonymisations in open-ended variables using [square brackets].
- Be as consistent as possible in anonymising data series to facilitate comparative examination.
- When anonymisation is finished, erase the original data files as well as any information regarding the anonymisation of open-ended responses in the syntax or other files that would disclose the original information.
- Review the background material relating to the data as they may also contain identifiers that must be erased or anonymised (research participants' contact information, paper questionnaires etc.).
In anonymising quantitative data, we want to eliminate exceptional observations that may increase disclosure risk. This is why it is recommended to examine the relationship between rare or unique observations and indirect identifiers. Usually a researcher should inspect all variables containing indirect identifiers or, ideally, all variables in the data. (Cabrera 2017.) You can search for rare or unique records, for example, by examining the categories and frequency distributions of variables with indirect identifiers. Cross tabulating variables may also be useful in finding exceptional cases and records. If there are continuous variables in the data, it is a good idea to recode them into categorical variables for disclosure risk assessment (ibid.). Continuous variables include, for instance, age or income when they can take on any real value on a continuum.
When cross tabulating variables, it is worth keeping in mind that categories with few observations do not necessarily always constitute identifying information. For example, if a survey is conducted in five schools with roughly the same number of pupils and only four pupils from one of the schools respond, these four observations are not automatically identifying information simply because of the small frequency count. This is because the potential number of respondents was as large as in the other schools. The situation would be different if this school had significantly fewer pupils than the others.
Anonymisation techniques
Anonymisation techniques for quantitative data can be divided into two categories: generalisation and randomisation. When data are generalised, information is irreversibly removed or attributes of data subjects are diluted by (re-)categorising or coarsening values, i.e. modifying their scale or order of magnitude. Randomisation techniques are used to add "noise" to the data to increase uncertainty of observations. (Cabrera 2017; EU's article 29 working group: Opinion 05/2014.) Successful anonymisation usually requires the use of several anonymisation techniques as well as assessment of the balance between data anonymity and data usability.
All anonymisation techniques have their advantages and limitations, which is why you should familiarise yourself with their effects on data quality and usability. Categorising variables enables retaining information in the data and utilising it with certain research methods. Categorisation lessens data usability, but only slightly (Purdam & Elliot 2007). In terms of anonymity, however, it is problematic that an entity can still be linked to a specific category after recoding (EU's article 29 working group: Opinion 05/2014). Moreover, categorising all values of a variable may make it difficult to determine relationships between variables and prevent the use of certain data analysis techniques designed for continuous variables (Anguli, Blitzstein & Waldo 2015).
Randomisation may be useful when there are relatively few rare observations in the data (under one percent). However, when using randomisation techniques, you should carefully assess the impact of the technique on the quality of the data. Randomisation techniques may have a significant effect on, for instance, the frequency distributions of variables and analyses of correlation and causation. These, in turn, affect research results. Although some researchers consider different randomisation techniques distortion of data, they are often useful in anonymisation.
In the following sections, we present the most common generalisation and randomisation techniques. Generalisation techniques include excluding, categorising and coarsening information, using samples instead of the whole data, and k-anonymisation and l-diversity. Randomisation techniques obscure the exact values of variables through multiplication and permutation.
Techniques:
- Removing variables, values and units of observation
- Recoding variable values
- Editing responses in open-ended variables
- K-anonymity and l-diversity
- Noise addition
- Permutation
1. Removing variables, values and units of observation
In the case of direct or indirect identifiers, removing a variable is the easiest and most obvious way to decrease the risk of identification. Naturally, variables containing indirect identifiers can also be removed when necessary. For instance, if the young participants of a survey on self-reported crime are asked which school they attend, the variable may present a disclosure risk when linked with other background variables. In this case, the school variable should be removed.
Sometimes it is also necessary to remove open-ended variables to prevent disclosure. This is often done when information in an open-ended variable is available in the data in another, categorised variable. For instance, if there is a categorised variable on the type of educational institution, the open-ended variable charting the names of the participants' educational institutions is removed. If exact information in an open-ended variable is crucial for research, one possible solution is to detach the variable from the data into a separate file. You can then coarsen the background variables you need for analysis and include them in the file. If linking the contents of the open-ended variable with the original data constitutes a disclosure risk, you should edit and organise the separate file in a manner that does not allow linking.
Removing individual values from records containing indirect identifiers may be justified if a value constitutes a disclosure risk, i.e. it is exceptional or rare. Such a value could be, for example, exceptionally high income or a rare occupation like minister (member of the government). When removing individual values, you should consider whether the removed information can be inferred by potential attackers. For example, the data of a total population study collected from workplace X contains the job titles of all employees and one title is only held by two people. Recoding this value as missing data would not be a good anonymisation solution, as it is relatively easy to find out the original job title. Instead of removing the value, a better solution would be to coarsen the job titles or combine some of the categories.
A whole data unit (individual, respondent) may be removed if it is not possible to otherwise remove identifying information on the individual. In some situations, this is a better option than using restricting techniques on the whole data only to de-identify one data unit.
2. Recoding variable values
Recoding the values of a variable is a better solution than simply removing the variable. For instance, instead of including the names of schools, you can recode the school variable into broader categories such as 'lower secondary school', 'upper secondary school', 'vocational school', etc. You can also categorise identifiers like exact age, municipality of residence and occupation. For instance, record the year of birth rather than the day, month and year, or recode it into categories that contain 3-5-year age groups.
Variables containing detailed geographical information, such as postal codes, can be aggregated from five-digit variables into two- or three-digit ones. The variable identifying the respondent's municipality of residence can be aggregated into two different variables: region/province and municipality type (urban, semi-urban, rural, etc.). This is a way to minimise identification risk without losing background information relevant for research. The social and regional classifications by Statistics Finland help in categorising variables.
- Social classifications of Statistics Finland (Opens in a new tab)
- Regional classifications of Statistics Finland (Opens in a new tab)
One way to reduce disclosure risk is to restrict the upper and lower ranges of a continuous variable to exclude outliers. This anonymisation technique is typically used for income variables. Highest incomes may be top-coded, that is, coded into a new category (e.g. "60,000 euros or over") while other income responses are preserved as actual values. In the same way, the smallest observed values can be bottom-coded.
Categorising or coarsening variables may significantly diminish the possibility to draw statistical conclusions. A good option for balancing between data utility and disclosure risk is to recode some values of a variable into broader categories discretionarily. If the frequency distribution is between 1-20 and most cases fall into values 1-12, it may be a good idea to leave the values under 10 as they are and combine the higher values into broader categories like 13-15 and 16-20. However, you should pay attention to the impact of this technique on the mean of the variable as well as on correlations between different variables.
Categorical variables have to be anonymised if one or more categories constitute a risk of identifying an individual. An identifiable category is combined with another category or multiple categories. To facilitate using the categories in analyses, the categorisation should be made according to some factor that connects the categories, if possible. For instance, if a category labelled 'civil partnership' requires anonymisation in a variable denoting marital status, the category should be combined with the category 'marriage', because a civil partnership and marriage are more similar and more useful in analyses than if 'civil partnership' were categorised together with the categories 'widow(er)' or 'single, never married'. It is also possible to recode identifiable categories as 'missing' but this should only be done if the variable already has enough missing observations so as to avoid the possibility of deducing the recoding of the category.
Another way to remove identifiers is to categorise open-ended responses. This technique functions well for open-ended questions collecting background information such as place of residence, education, educational institutions, place of work etc. For instance, a survey of physicians might contain an open-ended question on specialisation. Linked to other background variables, this variable might lead to an identification of physicians who are specialised in more than one area. One solution is to code the open-ended variable to have a broader category 'two or more areas of specialisation'.
It is also possible to change open-ended responses into a dichotomous variable (responded - did not respond) if the textual responses could lead to disclosure risk when linked to other background variables. This may be convenient for mainly quantitative variables where most response options have been classified and a separate open-ended 'Other, please specify' option has been created for responses that do not belong to any classes mentioned. For example, such a question may be used to ask what the participant's mother tongue is, with response options '1) Finnish, 2) Swedish, 3) Other, please specify' or to ask about religious denomination (Evangelical-Lutheran; Orthodox; Other, please specify). The open-ended responses given to the last alternative may constitute an identification risk when linked to other background variables. A good solution is to remove the open-ended responses from the data and only leave information on whether the respondent chose this option or not.
3. Editing responses in open-ended variables
Open-ended questions, which the respondents can answer in their own words, occasionally contain identifiers. These identifiers may relate to the respondents themselves or to third persons. The information in open-ended responses does not suffer decisively if identifiers (names, phone numbers, email addresses etc.) are removed. When it comes to other potentially identifying information in open-ended variables, disclosure risk should be assessed on a case-by-case basis taking into consideration the topic of the study and available background variables.
You can mark the anonymised names, words and excerpts in the data with square brackets. Original terms may be substituted by coarser, more general terms within square brackets or simply marked as [identifier removed]. In anonymising open-ended responses, you should consider whether the original value of the anonymised information can be easily determined due to its exceptional nature. For example, in a survey collected from all teachers in Anytown Elementary, one teacher says she works in the school's only special unit called Appletree, which has only three employees. Because there are very few teachers in the unit, the information is identifying and should be deleted, e.g. like this: [identifier removed]. Simply anonymising the special unit as follows is not sufficient: [special unit Y of Anytown Elementary removed]. This is because the unique special unit is easily inferred. All additional information in open-ended responses revealing that the teacher works in the special unit should also be removed. See instructions on how to anonymise qualitative data for further advice on anonymising open-ended variables.
4. K-anonymity and l-diversity
There are statistical anonymisation methods for assessing disclosure risk that help a researcher gain perspective on the anonymity of their data and justify the decisions made. One of the best-known of these methods is k-anonymisation, which is an attempt to combine the best features of statistical approaches to anonymisation (Elliot et al. 2016). K-anonymisation and l-diversity can be used, for example, when data are collected from a complete population and there are attributes that enable indirect identification of individuals or clusters of individuals. Such data include patient data, among others. K-anonymisation and l-diversity can also be used to ensure successful anonymisation after other anonymisation techniques have been used. There are free anonymisation tools available online, such as ARX (Opens in a new tab) and µ-ARGUS (Opens in a new tab) (ibid.).
K-anonymisation aims to prevent the identification of a data unit by forming a group of at least k records with the same attributes (El Emam & Dankar 2008). In other words, there should be at least k records in each value of a variable. For example, in a situation where a dataset contains only one male aged over a hundred years from Tampere, this individual should be grouped among others so that he is not the only person with these attributes. If the data contain other males over the age of 90 from Tampere, the hundred-year-old could be grouped among them. There is not an exact value for k and it should be decided on a case-by-case basis. Sometimes, a k of two data units may be sufficient (Cabrera 2017), but at least three is preferable. Some scholars have claimed that k should contain 5-10 data units. (Anguli et al. 2015; Machanavajjhala et al. 2007).
The problem with k-anonymity is that it does not prevent an attacker from inferring what kind of sensitive attribute is in question if all individuals of a k-anonymised group share the same value of the attribute. That is, k-anonymisation prevents identity disclosure but it does not prevent attribute disclosure This is where l-diversity becomes useful. L-diversity ensures that in a group of data units with identical attributes there are at least l values for a sensitive attribute. In other words, there should be enough variability between the values so that an attacker cannot infer what kind of sensitive information the value contains. (EU's article 29 working group: Opinion 05/2014.) It should be noted that l-diversity is not a de-identification technique per se but it prevents uncovering what kind of sensitive information pertains to an individual if the individual is re-identified (Cabrera 2017).
An example of l-diversity: data collected from all inpatients of an eating disorder clinic contain sensitive information on whether the respondent has tried to commit suicide in the past two years (yes/no). The respondents are k-anonymised into groups of at least three individuals in terms of certain indirectly identifying attributes (age group, gender, town of residence). This technique is sometimes called 3-anonymity (Cabrera 2017). When examining the sensitive information on suicide attempts, it becomes apparent that all male respondents aged 25-34 from Tampere have tried to commit suicide in the past two years. Therefore, if an attacker knows the identity of any male aged 25-34 from Tampere who had been an inpatient at the clinic during the survey, it is immediately obvious that this individual has tried to commit suicide. In order to achieve l-diversity (e.g. l =2), there should be both those who had tried to commit suicide and those who had not in the group. In an l-diverse group, automatically determining a suicide attempt based on the group is not possible. The term 2-diversity is sometimes used in a situation described above where the sensitive attribute has two distinct values (ibid.). Because l-diversity is not achieved in the example, one option would be to coarsen background variables (e.g. municipality of residence into region of residence).
T-closeness can be used if it is important to keep the data as close as possible to the original. T-closeness is achieved when there are at least l different values within each equivalence class and each value is represented as many times as necessary to mirror the initial distribution of each attribute. For more details on t-closeness, see, for instance, EU's article 29 working group: Opinion 05/2014.
5. Noise addition
Adding noise refers to modifying attributes in the data so that they are less accurate in order to increase uncertainty over the exact values of observation. Noise can be added in various ways. For example, values of the age attribute could be expressed with an accuracy of +/-2 years. An observer of the data will assume the values are accurate, although they are only so to a certain degree. Noise can also be added by multiplying the original values by a random number or by transforming categorised values into other values based on predetermined probabilities. An example of the latter would be transforming 15% of North Karelians into inhabitants of the Kainuu region. In addition, identifiable values of continuous variables may be aggregated into group means. Here one should ensure that each group receives a sufficient number of observations. (Cabrera 2017.) For instance, the exact drug costs of patients with sensitive illnesses could be replaced by the average drug costs of patients with these illnesses.
6. Permutation
Permutation refers to altering the values of variables containing indirect identifiers by swapping them from one record to another. By swapping the values between data units, the variance and distribution of a variable will not change, but correlations between the variable and the values of other variables for the particular individual are lost. Consequently, permutation should only be used with variables that are not strongly correlated. Permutation will not provide strong guarantees if two or more attributes have a logical relationship and they are permutated independently because an attacker might be able to determine the permutated attributes and reverse the permutation. (EU's article 29 working group: Opinion 05/2014.) For example, in a situation where two attributes, such as income and occupational status, have a strong logical relationship and one of them needs to be anonymised, consider using some other anonymisation technique instead of or in addition to permutation. Information anonymised using permutation may be determined based on correlation, and this increases the risk of de-anonymisation.
Anonymisation of qualitative data
Practical tips for anonymising qualitative data:
- At the planning stage, experiment with anonymisation by processing a couple of files at first.
- Back up the files to be anonymised and anonymise the copied files. This way, possible errors in anonymisation can still be fixed.
- Document the anonymisation process with a work document which should include, for instance, aliases used for personal names as well as categorisations that require consistency. Example: Interview 1: Simon=Matthew, Helsinki=[municipality 1].
- Use specific characters, such as [square brackets], for anonymisation to help keep track of what has been changed and what has not. Do not use text styling, such as italicised or coloured text, because these changes may disappear.
- Utilise 'find & replace' e.g. on Word to change names to their aliases. The command is also useful at the end of the anonymisation process when you review that all names have been anonymised. Be careful with the 'replace all' command, since names can appear as part of other words as well. For instance, the name 'Tim' is included in the word 'estimate'. If necessary, tick the 'match case' box so that the program will only replace sequences of characters that have the same case (Tim, not tim).
- When anonymisation is finished, erase original files and lists of aliases. Review the background material relating to the data, because they may also contain identifiers that must be erased or anonymised (research participants' contact information, paper questionnaires etc.)
- When transcribing interviews, mark each proper noun with a special character that is not used elsewhere in the text (e.g. #). This will make later anonymisation of names easier.
Anonymisation techniques
The techniques presented here can be applied to datasets as well as excerpts of data included in publications. The instructions only apply to textual data, such as transcribed interviews. We do not offer guidance on anonymising audio or video recordings.
The starting point in making a textual dataset anonymous is to erase background material containing identifiers, such as the contact details of participants and background information forms.
When you edit or remove identifying information, mark the changes clearly. You can use square brackets: [edited text], or double square brackets: [[edited text]].
Usually multiple of the techniques described below have to be utilised in anonymising an individual dataset.
Techniques:
- Replacing personal names with aliases
- Categorising proper nouns
- Changing or removing sensitive information
- Categorising background information
- Changing values of identifiers
1. Replacing personal names with aliases
Changing proper nouns into aliases is the most popular anonymisation technique used for qualitative data. However, aliases do not render the data anonymous until the original identifiers are completely disposed of. Research teams must be consistent in the selection and use of aliases throughout a research project. A spreadsheet file available to all team members can be used to maintain a list of names and their aliases. The same aliases should be used in both the data and the published excerpts.
When anonymising proper names, it is always a better option to use aliases rather than simply delete the names altogether or replace them by a letter or a character string, such as [x] or [---]. Replacing proper names with aliases makes it possible for the researcher to retain the internal coherence of the data. In cases where several individuals are frequently referred to, data may become unintelligible if the proper names are simply removed.
Using an alias for both the first name and the surname may be justified to make the transcription resemble natural speech or to keep a large number of participants separate from one another. The usual procedure, however, is to replace the first names with aliases and remove the surnames. If a person is referred to by his/her surname only, the alias should also be a surname.
A dataset may contain references to persons who are publicly known because of their activities in politics, business life or other work-related spheres. Their names are not changed. However, an alias or categorisation (e.g. [local politician]) should be used if the reference is related to the person's private affairs.
2. Categorising proper nouns
Names of persons who are mentioned in the text only once or twice and who have no essential importance in understanding the data content can be removed from the data without creating aliases. These names can simply be replaced with broader categories ([woman], [man], [sister], [father], [colleague, female], [neighbour, male] etc.). Using aliases is not always necessary for other proper nouns, either. If the data unit (personal interview, group interview, biography, letter, etc.) only discusses, for instance, one school or place of residence, its name can be replaced with a category, for instance, [lower secondary school], [home town] or [residential area].
Workplaces or other organisations that may constitute indirect identifiers in a dataset can be coarsened using Statistics Finland's Industrial Classification. Another possibility is to simply generalise e.g. Peters & Peters into [law firm], Tottenham Hotspur into [football club] and Pizza Hut into [restaurant].
Standard Industrial Classification of Statistics Finland (Opens in a new tab)
Locations appearing in the text can be coarsened by replacing them with e.g. [population centre], [city district], [village] etc. If you are not sure whether a place name is the name of a municipality or a district belonging to a municipality, place name directories or municipality catalogues may be of help.
If it has been decided that the participants' municipality of residence will not be revealed, researchers should remember to remove identifying geographical information relating to participants' place of residence both from background information and the textual data content. For instance, if the participant mentions that he or she often goes to a specific named restaurant that is located a short walking distance away from his or her home, it is best to replace the name of the restaurant with the generic expression [restaurant].
3. Changing or removing sensitive information
Identifying sensitive information should be removed, categorised or classified. For example, 'AIDS' could be changed to [severe long-term illness] and thereafter referred to as [illness], provided that the reader is able to deduce from the context that [illness] refers to the 'severe long-term illness' mentioned previously.
Removing or generalising sensitive data is justified if a) the respondent mentioned it only incidentally, b) the information is not relevant to the subject matter and c) the sensitive information constitutes a disclosure risk. For example, if a study focuses on the lives of persons with a severe illness, disclosure risk can be best reduced by using other anonymisation methods than altering crucial information.
4. Categorising background information
Background characteristics of participants, such as gender, age, occupation, workplace, school, or place of residence, are often essential for comprehending the data. Such characteristics may also constitute important contextual information for secondary analysis. Detailed background information can be edited into categories similarly to indirect identifiers in quantitative data. Various existing classifications, such as those used by national statistical institutes, are helpful in the process. If researchers create their own classifications, the classifications should be documented in detail in the data description.
Categorisation is often a better solution than deleting background information. An example: A 44-year old woman living in Tampere is interviewed for a study. She works as system specialist in the Computer Center at Tampere University, and she is married with two children aged 9 and 11. To reduce the risk of identification, her background information could then be categorised in the following manner:
- Gender: Female
- Age: 41-45
- Workplace: university
- Occupation: Information and communications technology (ICT) professional
- Household composition: Husband and two school-age children
- Place of residence: urban municipality in Western Finland
In the example above, the workplace (university) does not need not be generalised into [public sector employer], since the remaining background data do not allow even a partial identification. There are three universities and some separate units of other universities in the region of Western Finland.
When considering the need to categorise background information, researchers should take into account the other anonymisation techniques explained above as well as the subject matter and content of the data.
- Social classifications of Statistics Finland (Opens in a new tab)
- Regional classifications of Statistics Finland (Opens in a new tab)
- Industrial Classification of Statistics Finland (Opens in a new tab)
5. Changing values of identifiers
Sometimes it is possible to anonymise qualitative data by distorting information, just like values of identifying attributes can be swapped between records in quantitative data. For instance, an exact date of birth – which as an identifier should normally be removed – may sometimes be crucial for understanding the content.
A hypothetical example: The interviewee was born on 31st December 1958. On New Year's Eve in 2005 she sat by the hospital bed of her dying child. In the interview, she describes in detail her conflicting emotions evoked by the fact that New Year celebrations, the death of her child, and her own birthday are all mingled together in her mind.
In a case like this, deleting New Year's Eve from the data would prevent us from understanding the content. The date (New Year's Eve) can be retained in the data if the interviewee's year of birth is changed to one or two years earlier or later.
6. Removing hidden metadata from files
During anonymisation, it is important to check whether archival files contain any hidden technical metadata that could enable the identification of research participants. This hidden metadata consist of, for example, location information and information about the owner of a device or a user profile. Technical metadata may be saved when files are created but also when they are edited.
Research data in the form of text or images may consist of files created by the research participants themselves. Risk of identification from the metadata is particularly high in these cases. As textual data often comprise text files created and directly submitted by the research participants, the hidden metadata of these files refer to the participants explicitly. EXIF data of digital images may also contain very precise information, such as the exact coordinates of where the picture was taken and even the photographer's name.
Technical metadata can be removed by using common text or picture editors (e.g. MS Office, Windows File Explorer, Photoshop, GIMP, Irfanview). There are also programs specifically designed for removing EXIF data (e.g. Easy Exif Delete), which make removing the hidden metadata easy. Specific instructions on how to remove technical metadata depend on the software and its version. See the instructions on the website of the program you are using.
Identifier type table
Different types of identifiers are listed in the table below. Information that belongs to the special categories of personal data specified in the GDPR is marked with an asterisk ( * ). Each identifier is characterised as either direct identifier, strong indirect identifier or indirect identifier.
The last column notes the easiest methods for dealing with that type of identifier. The methods include removing the identifier, changing it into an alias and categorising or classifying it.
Some attributes may be both indirect identifiers and strong indirect identifiers. For example, an unusual occupation or occupational status is a strong indirect identifier, while a common occupation is an indirect identifier.
The table is not exhaustive but may provide good tips for recognising identifiers and anonymising research data.
Skip tableIdentifier type | Direct identifier | Strong indirect identifier | Indirect identifier | Anonymisation method |
---|---|---|---|---|
Social security number | x | Remove | ||
Full name | x | Remove/Change | ||
Email address | x | x | Remove | |
Phone number | x | Remove | ||
Postal code | x | Remove/Categorise | ||
District/part of town | x | Categorise | ||
Municipality of residence | x | Categorise | ||
Region | x | (Categorise) | ||
Major region | x | |||
Municipality type (urban, semi-urban, rural) | x | |||
Audio file | x | Remove | ||
Video file displaying person(s) | x | Remove | ||
Photograph of person(s) | x | Remove | ||
Date/year of birth | x | Categorise | ||
Age | x | Categorise | ||
Gender | x | |||
Marital status | x | |||
Household composition | x | (Categorise) | ||
Occupation | (x) | x | Categorise | |
Industry of employment | x | |||
Employment status | x | |||
Education | x | Categorise | ||
Field of education | x | |||
Mother tongue | x | Categorise | ||
Nationality | x | (Categorise) | ||
Workplace/employer | (x) | x | Categorise | |
Vehicle registration number | x | Remove | ||
Title of publication | x | Categorise | ||
Web page address | (x) | x | Remove | |
Student ID number | x | Remove | ||
Insurance number | x | Remove | ||
Bank account number | x | Remove | ||
IP address | x | Remove | ||
Health-related information * | (x) | x | Remove/Categorise | |
Ethnic group * | (x) | x | Remove/Categorise | |
Crime or punishment | x | Remove/Categorise | ||
Membership in a trade union * | x | Categorise | ||
Political or religious allegiance * | x | Categorise | ||
Other position of trust or membership | (x) | x | Remove/Categorise | |
Need for social welfare | x | Remove/Categorise | ||
Social welfare services and benefits received | x | Remove/Categorise | ||
Sexual orientation * | x | Remove |