Processing Qualitative Data Files
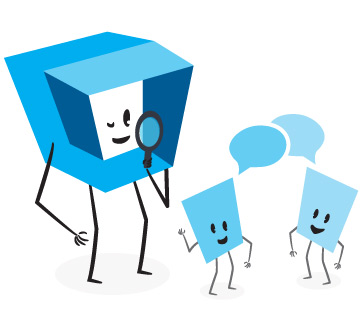
Qualitative research data may consist of many different types of research material. These may include transcribed interviews, written texts, still images, ethnographic diaries and audio and video recordings.
The qualitative data archived at the Finnish Social Science Data Archive (FSD) are mainly textual. Textual data may include, for example, anonymised and transcribed interviews and various types of written texts. Additionally, digital photographs can be archived at FSD if they are used as empirical data in research, do not contain identifying information, and the photographer has given consent for archiving (see Agreement with external parties concerning the transfer of copyright and ownership rights (Opens in a new tab) ). The FSD has made an agreement with the Finnish Copyright Society Kopiosto which allows the FSD to archive and disseminate copyrighted data collected by researchers, such as newspaper and magazine articles, photographs, cartoons and illustrations in books.
The FSD does not generally archive audiovisual material. In some cases, short video clips used as a tool in data collection and video invitations to participate in research may be archived. Audiovisual material are mainly archived and disseminated for further research by the Language Bank of Finland (Fin-CLARIN). If you are planning to collect audiovisual material in your research or already have such material that you wish to archive for data sharing, contact the Language Bank.
Language Bank of Finland, coordinated by FIN-CLARIN
(Opens in a new tab)
As a rule, only anonymised transcriptions of interview data are accepted by FSD for archiving, and audio recordings of interviews are not archived. However, audio recordings of interviews may in some cases be archived (e.g. certain kinds of expert interviews), if the interviewees have given written consent for the archiving of the interviews with their personal data.
In order to help researchers in data management during their research process and to facilitate data reuse, the FSD provides examples below on various qualitative data processing methods.
Transcription
The most common formats of qualitative data are written texts, interview data and focus group discussion data. In most cases, interview and discussion data are first digitally recorded and then transcribed. Representing audiovisual data into written form is the most typical way of processing interview and discussion data into an analysable format. Occasionally, the recordings themselves are analysed, for instance, in studies focusing on language or interaction.
The FSD archives interview and discussion data that are already transcribed. The level of transcription is always decided by the original researcher or research team and is dependent on the objectives set for the data. Transcription level decisions are often influenced by the resources available. In an ideal case, researchers understand how valuable the data may become for other researchers outside the original research team and thus allocate resources to the transcription. It is recommended that transcription of the recorded material is done as extensively as possible. As it is hard to say in advance for what kind of study the data will be used in future, it is often best to also transcribe those parts that do not seem relevant at the time. This also enables the researchers themselves to reuse the data later for other new research questions.
It is advisable to consider the future archiving of the data and the potential need for anonymisation already when planning the transcription of recorded material. Moreover, research grant applications should cover funding for the purpose of planning and carrying out anonymisation in addition to transcription. Nowadays, research funders often recommend or even require the data they funded to be archived, and anonymisation is one of the prerequisites for archiving research data. Anonymisation is often easiest to carry out at the same time as the transcription of the material, especially if the anonymisation has been carefully planned ahead (see guidelines for anonymisation ). The required level of anonymisation depends on how the research participants have been informed about the use and processing of the data (see guidelines for informing research participants ). Researchers can do the transcription themselves or buy it from a service provider. The FSD does not provide transcription services.
There are no established names or definitions for different levels of transcription, although there is some agreement on the general guidelines. In practice, transcription does not follow any particular level but combines features from different levels, tailoring the transcription to the requirements of the material at hand. Whatever the level chosen, it is essential to be uniform and consistent throughout in the level of detail and logics of transcription.
Different levels of transcription can be classified in a following manner, for instance:
-
Gisted/summary transcription:
Interview recordings are represented into written form only roughly, by listing or summarising main points/topics. Direct quotations or parts of speech are only rarely written down. Interpretation plays a big role in this kind of transcription because it is the transcriber who decides which parts are worth transcription.
→ Can be used, for instance, for producing articles based on interview data. Does not enable in-depth analysis nor does it support rich and varied use and reuse of the data. -
Basic level transcription:
Will produce a verbatim (exact) transcription of utterances but leaves out repeats, cut-offs of words and sentences, fillers ('you know'), and non-lexical sounds ('uh', 'ah'). Utterances clearly not in context can also be left out. In addition to speech, significant expressions of emotion (laughter, getting upset etc.) are incorporated.
→ Can be used when the main focus is analysing the content of speech. This is the minimum transcription level for data sharing and archiving. -
Exact transcription:
All speech is transcribed, nothing is left out. Transcription is a verbatim, word-for-word replication of the verbal data, using the most common standardized notation symbols. Fillers ('you know'), repeats, cut-offs of words and non-lexical sounds are incorporated in the transcription, as well as expressions of emotion (laughter, sighs, getting upset etc.) and emphasis or stress. Timed pauses (in seconds) and possible background noises and other disturbances are noted .
→ Often used when there is intention to analyse expressions and interaction, at least to some extent. This level of transcription allows for varied and rich reuse of the data. -
Conversation analysis transcription:
Full verbal transcription using standardized notation symbols, with careful reproduction of colloquial speech patterns. Transcription includes all words, timed pauses (in seconds), cut-offs of a word, intonation, volume, word stress, as well as non-lexical action (sneezes, breaths, sighs, facial expressions) etc.
→ The most detailed level of transcription. The goal is to represent the conversation event in as much detail as possible in textual format. Often used together with the audio and video recordings themselves.
Both for the sake of one's own research and for the sake of data reuse, it is always better for transcription to be too detailed than vice versa. If interview records have been represented into text only in a summary format, this may become a problem even for the original researchers at the analysis stage. The minimum transcription level for data reuse and sharing is basic level transcription. Data reuse is further enhanced if exact transcription has been used. Whether a yet more detailed transcription level is chosen is dependent on the research objectives and resources available.
If transcription notation symbols are used, it is good to remember that the symbol signs of word processor programs may change when converted to other software programs. Formatting, footnotes and links to other documents may also disappear in conversion. It is therefore advisable never to enter content or structural information using formatting (i.e. using bold, italics, underline, colours, indent etc.). It is safest to use only the symbols available in keyboards. It is safest to use only the symbols available in keyboards.
The notation symbols used in transcription should be described in interview guidelines and consequent data documentation. This way the same notations will be used systematically and consistently throughout. Having information on the notations used in transcription is essential for data reuse, or when data are collected and transcribed in different locations, and even in cases when it is the original researchers who are reusing the data, because memory is short. Without notation information, it soon becomes impossible to understand what each symbol means. When a standardised notation is used, it may be enough to enter a detailed reference to the original source of the notation.
Speaker demarcation should be consistent throughout the transcription to facilitate readability and to allow for automatic processing at some stage. Each time the person speaking changes, his or her speech should be transcribed as a discrete unit, always starting from a new row. For instance, at the beginning of the row, a speaker ID is entered, followed by colon (:). The speaker ID may be the name of the speaker, initials of the name or a pseudonym, as long as they are used consistently.
For instance,
Interviewee 6: I'm guessing they did, for most part.
Interviewee 7: Oh, yes, I thought so as well.
Organising and naming data files
When the data have been collected, saved and possibly transcribed, it is time to decide how to organise the storage of the data and name the data files. Systematic and consistent organisation and naming of data files facilitates data management during research as well as data archiving and reuse. The decision on how to organise and name the data files should be made on a case-by-case basis.
All material relevant to the data should be entered into the data folders. Data files should be stored in a format that is commonly used and supported by several different software so that the processing and later archiving of the data can be conducted without problems (see suitable file formats ). It is good to remember that data do not consist only of the collected research data, but also the descriptive information on data collection and data processing procedures. Examples of relevant material include
- invitation to participate in research
- research information sheet for the participants
- consent form for archiving
- interview frame
- description of notation symbols used in transcription
- description of anonymisation
- possible stimulus material
Depending on the amount of data, one data file can include one or more data units (e.g. transcribed interviews or written texts). In most cases, it is advisable to store each data unit in a separate data file in the main folder (data folder). This way, one data file contains one data unit, such as a transcribed interview (see Example 1).
Example 1
-
Interviews on Bicycle Commuting 2018
-
-
Transcribed interviews
-
-
Interview_01.odt
-
Interview_02.odt
-
Interview_03.odt
-
Interview_04.odt
-
Interview_05.odt
-
Interview_06.odt
-
Interview_07.odt
-
Interview_08.odt
-
-
-
-
Information on data collection
-
-
Interview_frame.odt
-
Invitation_to_participate.odt
-
Consent_for_archiving.odt
-
Description_of_anonymisation.odt
-
-
However, sometimes storing the data units in separate data files is not practical. For instance, if the data contain several short texts (e.g. just a few lines), it might be more convenient to store all data units in one data file (see Example 2).
Example 2
-
Finnish Proverbs 2013
-
-
Proverbs 137pcs.odt
-
Data_collection_form.odt
-
Invitation_to_participate.odt
-
Consent_for_archiving.odt
-
Naming the data files in a descriptive manner that indicates whether the file contains a transcribed interview, written text or image facilitates data management and makes the files easier to distinguish and find. However, the names of data files should not include background information or other metadata. During the archiving process, the names of files and file formats are converted automatically to correspond to the conventions of the FSD. During this process, all information stored in the file names by the researchers will disappear. Additionally, if the background information appearing in the file name is coded too concisely, it may be difficult or downright impossible for outsiders or even for the original researchers to interpret them. It is recommended that the background information of research subjects is primarily entered in the beginning of each data unit, for example the beginning of a transcribed interview. This also better facilitates the reuse of the data. For photograph or newspaper data, the background information can be stored in a separate background data list (see entering background information into data files ).
Systematic naming of data files is especially useful when several different types of data files (e.g. audio tapes, their transcriptions and photographs taken by the interviewee) are connected to one data collection event (e.g. one interview). It is advisable to store each type of data file in their own folder and to connect the files that are related to one collection event through consistent and carefully considered file names. For instance, it is easy to deduce that two files named ‘Interview1.mp3’ and ‘Interview1.odt’ are an audio file and a transcription from the same interview. However, it should be noted that the archiving of audio recordings of interviews at FSD is possible only in some exceptions (e.g. certain kinds of expert interviews), and only if the interviewees have given written consent for the archiving of the interviews with their personal data (see Informing Research Participants about the Processing of their Personal Data ). The example below illustrates how the connection between photographs and interviews can be included in a separate background data list (see Example 3).
Example 3
-
City Architect Interviews 2019
-
-
Transcriptions
-
-
Interview_01.odt
-
Interview_02.odt
-
Interview_03.odt
-
Interview_04.odt
-
-
Audio tapes
-
-
Interview_01.mp3
-
Interview_02.mp3
-
Interview_03.mp3
-
Interview_04.mp3
-
-
Photographs
-
-
photos_background_data_list.odt
-
Photo_01.jpg
-
Photo_02.jpg
-
Photo_03.jpg
-
Photo_04.jpg
-
Photo_05.jpg
-
Photo_06.jpg
-
-
Data collection documents
-
-
Interviewframe.odt
-
Invitation_to_participate.odt
-
Consent_for_archiving.odt
-
-
In cases where several clearly independent sets of data (e.g. a separate questionnaire and interviews, data from different target groups) have been collected for a research project, it is recommended that a data folder is created for each independent set of data separately. During the archiving process, such independent data are mainly archived as separate datasets that can still be easily connected to each other.
Documenting background information
Data units (e.g. transcribed interviews, newspaper clippings, photographs, written texts) are always accompanied by some background information that makes the data useful for the researcher. In most cases, background information also has a significant role when analysing the data. As such, systematic storing of background information is an essential part of data management and is useful for both the original researchers who collected the data as well as for anyone who wants to reuse the data. When the background information in a dataset has been collected with consideration and recorded according to guidelines, the dataset keeps its value for further research even after primary use.
Background information relating to a data unit may include, for instance, information on the research subjects and the data collection event, and notes of the researcher. Background information on research subject may include, for example, gender, age group, occupation and education. Information relating to the collection event may include time and location of the interview and name of the interviewer, among other possible information on the event.
What background information is entered for each unit varies from data to data and is ultimately a decision of the original researchers. However, it is good to remember that recording background information which does not seem very relevant for the ongoing research may be of great importance in future when the data are reused for other research purposes. It is therefore better to record too much background information than too little. Removing superfluous information is always easier than complementing insufficient information. However, although background information should be as informative as possible, it is also important to keep in mind the consent given by research participants regarding their personal data, i.e. the type and level of identifying information that can be saved during research according to the agreement between the researchers and participants. One should also bear in mind that the EU General Data Protection Regulation (GDPR) prohibits collecting unnecessary personal data (see minimisation ). In planning what background information to collect and when categorising the collected data, you can refer to the categorised list of background information examples provided by the FSD.
Below are two examples of recording background information in a manner that facilitates data archiving and ensures that the information is retained in the processing of data. For text files (e.g. transcribed interviews and written texts), it is recommended that background information is entered in the beginning of data files. For other file types (e.g. newspaper articles, photographs, PDF documents) it is recommended that background information is stored in a separate file.
Entering background information into data files
When a textual dataset is archived at FSD, the archive produces a separate HTML index for the dataset, which allows for easier handling of individual interviews, written texts etc. The index enables users to easily identify and locate data units according to particular background information, for example, gender, age, or occupation. Additionally, the index enables targeted word searches for the contents of both the data and the index. To make the HTML index creation possible, it is important that background data fields can be parsed automatically for each data unit. For automatic parsing to be successful, it is particularly important that background information is systematically entered in the beginning of each data unit (e.g. interview transcript) in a standardised and uniform manner.
Example of HTML index produced by FSD for a textual dataset (Opens in a new tab)
Example 4 presents a typical transcript of an interview with only one interviewee. In this example dataset, the long transcripts of the interviews have been saved in separate files in a format that is commonly used and supported by several different software (e.g. ODT, TXT, DOCX. See Organising and naming data files, Example 1). Background data are entered in the following manner in the beginning of the first page of each transcription file.
Interview date: 08.02.2013 [=8 February 2013]
MM: First I would like to ask you about your choice of profession. How did it come about that you decided to become a teacher?
|
Example 5 is otherwise similar to example 4 expect that it is a focus group interview, with several interviewees. Therefore, each interviewee has an ID (e.g. R1, R2) which helps to identify their speech. The background information of each interviewee can be entered to the background data fields in the following manner. Other types of ID, such as a pseudonym, can also be used. If a pseudonym is used, it should be included in the background information as its own data field. Whatever ID system is chosen, it should be used consistently throughout the data.
Interview date: 08.02.2013 [=8 February 2013]
MM: First I would like to ask you all about your choice of profession. Tell me a bit about how you came to have the profession you have now?
|
In Example 6, research subjects were asked to write down one proverb that had been significant in their lives. Altogether, the data contain over 40 pages of proverbs provided by over 100 individuals. As the proverbs are short but the data as a whole quite large, it is easiest to store all proverbs in one file (see Organising and naming data files, Example 2). In cases like this, background data fields are entered in the beginning of each proverb so that they allow for automatic processing of data and the creation of an HTML index.
Occupation: Teacher
|
Occupation: Carpenter
|
Occupation: Journalist
|
Occupation: Software programmer
|
Automatic processing of data is possible if the background data fields (e.g. ‘Date of interview:’, ‘Interviewee's age:’) are created in an identical manner throughout the data and the fields are always in the same order. A very good way is to end the title of each background information field with a colon, followed by an empty space. Each background data field ends in a line break ('enter'), so it can be separated from other text. To avoid spelling mistakes and ensure that the order of the data fields remains consistent, it is easiest to copy the background data field titles as empty in the beginning of each text unit, that is, each proverb in our example case. Then all that remains is to enter the actual background information to the fields themselves for each subject.
Interviewee's gender:
Interviewee's occupation:
Interviewee's age:
Interviewer:
Storing background information in a separate file
For some types of data, the file format does not allow recording background information in the beginning of the data file. This is the case for photographs, audio recordings and protected PDF files, for example. In these cases, the best practice is to store background information in a manually created data list or a separate text file, which contains key background information for each data unit on successive rows. Systematic entering of background information in a data list or in a separate text file will facilitate data management in different stages of the research, as well as preserve collection event information that is important for later archiving and reuse of the data.
In a manually created data list, the background information is entered in a table using, for instance, Excel or the Open Office Calc program (see Example 7). If a separate text file is used, a machine-readable HTML index is not created until during the archiving process. The index enables easier handling and browsing of data files and related background information. To allow for the automatic creation of the HTML index, the optimal solution is to enter the background data fields in a consistent and uniform manner (see Example 8).
In both cases, background data contain the file names and background information on the data units. A data list for audiovisual data may also contain technical information connected to the collection event, such as the type and model of the device used for recording and the length of the video/audio etc. However, most technical background information can be read automatically from the audiovisual files themselves, so it may not be necessary to enter them manually into the background information.
Example 7 portrays a manually created background data list in Excel format. The data collected are photographs of writings or stickers on walls within public view in two Finnish cities. The data list contains background information on the date when the photograph was taken, the photographer, the place where the photograph was taken and a description of the photograph.
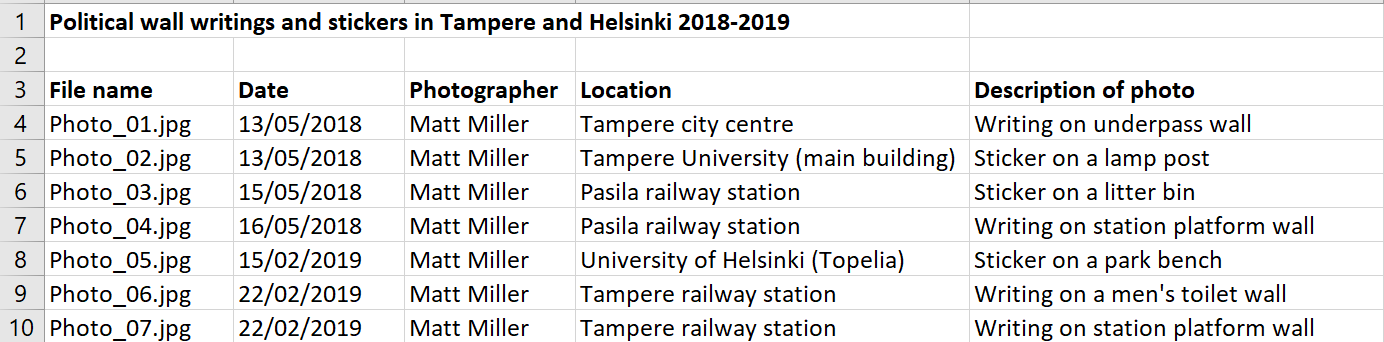
In Example 8, the background information has been entered into a separate text file, unit by unit. The background data fields are the same as in examples 5, 6 and 7. When they are entered in a separate text file as in the example here, the file name is included as the first background data field, linking them to the right unit. Each background information field should again end with a colon, followed by an empty space. Each entity of background information should be separated by at least one line break [enter].
File name: Photo_01.jpg
|
File name: Photo_02.jpg
|
File name: Photo_03.jpg
|
File name: Photo_04.jpg
|
File name: Photo_05.jpg
|
Data collected from periodicals
The FSD has made an agreement with the Finnish Copyright Society Kopiosto which allows the FSD to archive and disseminate copyrighted data collected by researchers, such as newspaper and magazine articles, photographs, cartoons and illustrations in books (see Member organisations of Kopiosto (Opens in a new tab) ). The FSD only accepts digital data that have been collected for research purposes.
Material collected from online periodicals
When researchers collect articles from online periodicals for research purposes, they should bear in mind that references to web resources, like URLs, may change over time. Because of this, articles deposited at the Data Archive for archiving should be copied into a word processing program. If the copied articles do not contain bibliographic information, it should be added in the beginning of the article. After this, it is advisable to convert the articles into PDF file format.
Documenting bibliographic information
When articles, photographs and other similar material are collected from periodicals for research purposes, bibliographic information should be carefully detailed. For example, bibliographic information of newspaper articles should include
- Author(s)
- Title of the article
- Title of the newspaper
- Date of publication
- Web address of the article (if online newspaper)
- For an online newspaper, retrieval date of the article
- If the text in question is an editorial, opinion piece or letter to the editor, this should be mentioned in the citation.
Examples of articles with a known author:
- Jamie Doward: Doctors told to curb overuse of oxygen in hospitals. The Observer 15.5.2016.
- Elif Shafak: Turkey wants to be less European, not more. The Financial Times 3.6.2016. Opinion.
- Warren Wilson: Don’t celebrate obesity. The Washington Times 26.5.2016. Letter to the editor. http://www.washingtontimes.com/news/2016/may/26/letter-to-the-editor-dont-celebrate-obesity/ (Opens in a new tab) . Retrieved 7.6.2016
Examples of anonymous articles:
- Food deliveries on the rise. YLE 1.6.2016. http://yle.fi/uutiset/food_deliveries_on_the_rise/8924161 (Opens in a new tab) . Retrieved 10.6.2016.
- EU referendum: MPs call for extended vote registration. BBC 8.6.2016. http://www.bbc.com/news/uk-politics-eu-referendum-36476176 (Opens in a new tab) . Retrieved 13.6.2016.
If the data under study includes articles from scientific journals, bibliographic information should also include, following the established citation conventions of academic writing,
- Page numbers of the article
- Title of the journal
- Journal volume number
- Journal issue number
If the articles have been collected from edited books, the bibliographic information should include
- Name(s) of the editor(s)
- Complete title of the book
- Page numbers of the article
- Title of the publication series, number of the edited book in the series
- Name of the publisher
- Place of publication
Make a list of analysed articles
A researcher planning to deposit articles collected from periodicals for archiving should deliver the Data Archive a separate listing of all the articles. The list should consist of bibliographic information sorted alphabetically or chronologically. Alternatively, the articles may be listed in the order the articles were analysed during research. The important thing is that the bibliographic information is documented consistently. The Archive delivers the list of articles to Kopiosto during the archiving process.
Digital photographs of archived paper records
The data utilised in humanities research have often already been archived as paper records, which are stored by the National Archives of Finland as well as other archives. In some cases, FSD may archive digital photographs of data that have previously been archived as paper records elsewhere. The FSD has made an agreement with the National Archives of Finland which allows the FSD to archive digital photographs of paper records stored by the National Archives if the photographs have been taken by the researcher for research purposes.
Digital photographs taken by the researcher of the paper records stored by the National Archives can be archived at FSD according to the following conditions:
1. The data archived at FSD must not already be digitally archived at the Digital Archives of the National Archives.
2. The digital photographs must have been taken to be analysed as research data.
3. The appropriate bibliographical information relating to the digital photograph must be included.
The bibliographical information should be included for each photograph in a similar manner to a corresponding paper record. Depending on the document, bibliographical information may include, for example,
- title of archive/fonds (i.e. authority, community, individual) or collection
- title of archive series
- year of archive item
- number or some other reference code of archive item
- the archive storing the document
- municipality
- selection criteria and explanation if only part of the document has been photographed
Examples of referencing digital photographs of archived paper records:
- SN-Seura Annual Report 1944, page 11, National Archives of Finland (KA)
- Turku and Pori Province Infantry Regiment, arrived letters 1723-1811, letter 12.11.1799, National Archives of Finland (KA)
Archiving digital photographs in practice:
Save each photograph so that its name illustrates the bibliographical information of the source material. For example, a digital photograph of a document containing an annual population census (henkikirja in Finnish) from the Viipuri Province from 1823, which has been archived as a paper record at the National Archives of Finland, can be saved as Henkikirja_VI_1823_KA.jpg. If an archive item consists of several successive photographs, the names should include consecutive numbering, i.e. Henkirja_VI_1823_KA_01.jpg, Henkirja_VI_1823_KA_02.jpg, etc.
Deliver the photographs to FSD as JPG files with a separate list that contains the bibliographical information of the archived source material of each photograph.
If your digitised materials fulfil the quality requirements of the National Archives of Finland, you can offer your data to be archived at the Digital Archives of the National Archives of Finland.